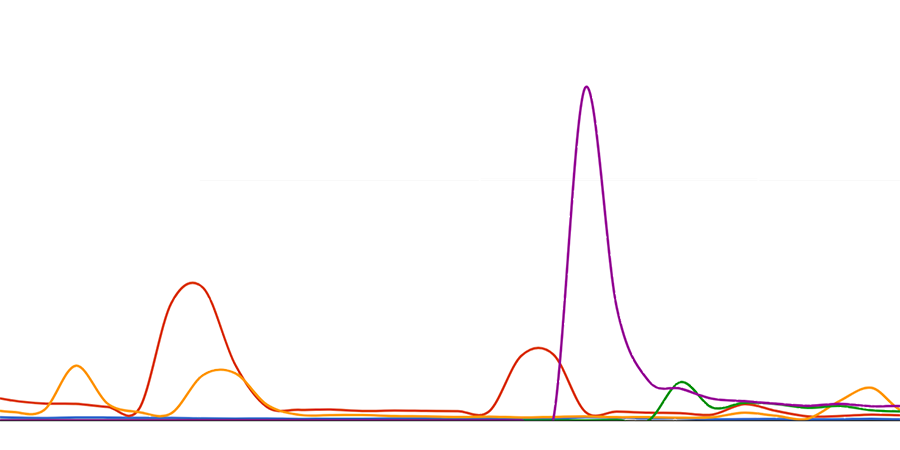
I took a statistics class in college, but I didn’t pay much attention due to never seeing a need for the knowledge. Much of my education is like this: “Why the hell would I ever need this”, only to find out years later that I desperately needed it (yeah, I’m talking to you, high school algebra).
Thimbleweed Park is out on seven different digital stores and each of them has a completely different way of reporting sales. It’s time-consuming and frustrating to download each sales report once a month and hand massage the data into a useable format.
I spent the last few days writing a python script to take all the data and move it into a single SQL database so I could quickly query tons of useful information.
@@pre@@ SELECT countries.country, SUM(sales.units_sold) AS units_sold FROM sales JOIN countries ON sales.country_code = countries.country_code WHERE units_sold > 0 GROUP BY sales.country_code ORDER BY units_sold DESC @@pre@@
One thing I want to know is an “average” units/day for each store, so I can keep an eye on long-term trends. Sounds easy, except for sales and promotions happening all-to-often, and they produce huge spikes in the data that I don’t care about. I just want to see an average for average non-sale days and I don’t want to go in and remove the sale ranges as sometimes they happen without my knowledge.
I’m looking for a way to take an average that removes spikes that are well above the average. This doesn’t have to be in SQL, that computation will most likely happen in a spreadsheet, or could happen in the report generation if it’s too complex.
Maybe this isn’t possible, but I figured I’d ask.